Utilizing Machine Learning to fortify sales cycle
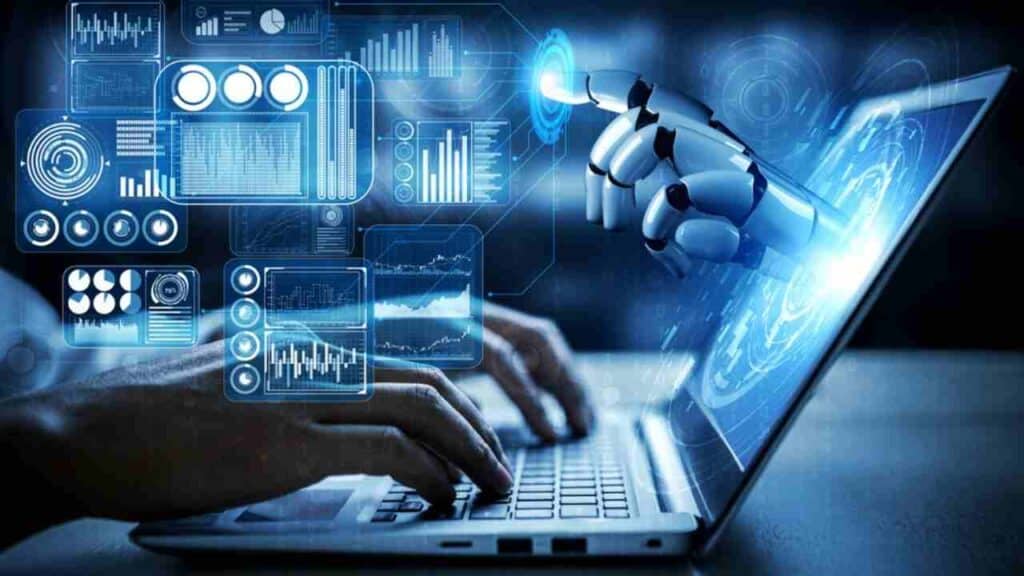
Machine learning has revolutionized various industries, and the sales sector is no exception. With its ability to analyze vast amounts of data and make accurate predictions, machine learning has become an invaluable tool for businesses looking to optimize their sales processes. In this article, we will explore how machine learning can be utilized to fortify the sales cycle, providing valuable insights and examples along the way.
Understanding the Sales Cycle
Before delving into the role of machine learning in the sales cycle, it is essential to have a clear understanding of what the sales cycle entails. The sales cycle refers to the series of steps a customer goes through before making a purchase. These steps typically include:
- Prospecting: Identifying potential customers
- Qualification: Determining if a prospect is a good fit
- Needs Analysis: Understanding the prospect’s needs and pain points
- Presentation: Presenting a solution to the prospect
- Objection Handling: Addressing any concerns or objections the prospect may have
- Closing: Converting the prospect into a paying customer
- Follow-up: Maintaining a relationship with the customer and ensuring their satisfaction
The Role of Machine Learning in the Sales Cycle
Machine learning can play a significant role in each stage of the sales cycle, enhancing efficiency and effectiveness. Let’s explore how machine learning can be utilized in each step:
1. Prospecting
Identifying potential customers is a crucial step in the sales cycle, and machine learning can assist in this process. By analyzing vast amounts of data, machine learning algorithms can identify patterns and characteristics of ideal customers. This allows sales teams to focus their efforts on prospects who are more likely to convert, saving time and resources.
For example, a sales team can use machine learning algorithms to analyze customer data and identify common traits among their most valuable customers. This information can then be used to create a customer profile, which can be used to target similar prospects in the future.
2. Qualification
Qualifying prospects is another critical step in the sales cycle. Machine learning can help streamline this process by automatically scoring leads based on various factors such as demographics, behavior, and engagement. By assigning a lead score, sales teams can prioritize their efforts and focus on leads that are more likely to convert.
For instance, a machine learning algorithm can analyze a prospect’s online behavior, such as website visits, email opens, and social media interactions, to determine their level of interest. This information can then be used to assign a lead score, indicating the prospect’s likelihood of making a purchase.
3. Needs Analysis
Understanding the needs and pain points of prospects is crucial for providing tailored solutions. Machine learning can assist in this process by analyzing customer data and identifying common pain points and preferences. This information can then be used to personalize sales pitches and address specific needs.
For example, a machine learning algorithm can analyze customer support tickets and identify common issues faced by customers. This information can then be used to train sales representatives on how to address these pain points effectively.
4. Presentation
Presenting a solution to prospects requires effective communication and understanding of their preferences. Machine learning can help sales teams by analyzing customer data and providing insights on the most effective communication channels and messaging.
For instance, a machine learning algorithm can analyze customer interactions on different channels, such as email, phone calls, and social media, to determine which channel and messaging style yield the highest response rates. This information can then be used to tailor sales presentations to maximize engagement and conversion rates.
5. Objection Handling
Addressing objections and concerns is a crucial part of the sales process. Machine learning can assist in this step by analyzing customer data and identifying common objections. This information can then be used to train sales representatives on how to effectively handle objections and provide satisfactory responses.
For example, a machine learning algorithm can analyze customer feedback and identify common objections raised by prospects. This information can then be used to create a knowledge base of objection handling techniques, which can be accessed by sales representatives during customer interactions.
6. Closing
Closing deals is the ultimate goal of the sales cycle, and machine learning can help increase conversion rates. By analyzing historical sales data, machine learning algorithms can identify patterns and factors that contribute to successful closures. This information can then be used to optimize the sales process and increase the likelihood of closing deals.
For instance, a machine learning algorithm can analyze past sales data and identify common characteristics among customers who made a purchase. This information can then be used to create a scoring system that predicts the likelihood of a prospect converting into a paying customer.
7. Follow-up
Maintaining a relationship with customers is essential for long-term success. Machine learning can assist in this step by analyzing customer data and providing insights on the most effective follow-up strategies.
For example, a machine learning algorithm can analyze customer behavior, such as repeat purchases and engagement with marketing materials, to determine the most effective follow-up frequency and messaging. This information can then be used to personalize follow-up communications and increase customer satisfaction.
Case Studies and Statistics
Several case studies and statistics highlight the effectiveness of utilizing machine learning in the sales cycle:
Case Study: Company X
Company X, a software-as-a-service (SaaS) provider, implemented machine learning algorithms to optimize their sales cycle. By analyzing customer data and identifying patterns, they were able to streamline their prospecting process and focus on high-value leads. As a result, their conversion rates increased by 20% within six months.
Statistics: Industry-wide Impact
- A study by McKinsey found that companies that effectively utilize machine learning in their sales processes experience a 10-15% increase in sales.
- According to Salesforce, 51% of high-performing sales teams use AI and machine learning to personalize customer interactions.
- A survey by InsideSales.com revealed that 62% of sales representatives believe that AI and machine learning will have a significant impact on their ability to meet sales quotas.
Summary
Machine learning has the potential to revolutionize the sales cycle by providing valuable insights and optimizing various stages of the process. From prospecting to follow-up, machine learning can enhance efficiency, increase conversion rates, and improve customer satisfaction. By leveraging the power of machine learning, businesses can fortify their sales cycle and gain a competitive edge in today’s rapidly evolving market.
Visit https://SaasExpert.ca – Your All-In-One Sales and Marketing Platform for small businesses, agency owners, and marketers to learn more about how machine learning can transform your sales processes.
Frequently asked questions about Utilizing Machine Learning to fortify sales cycle
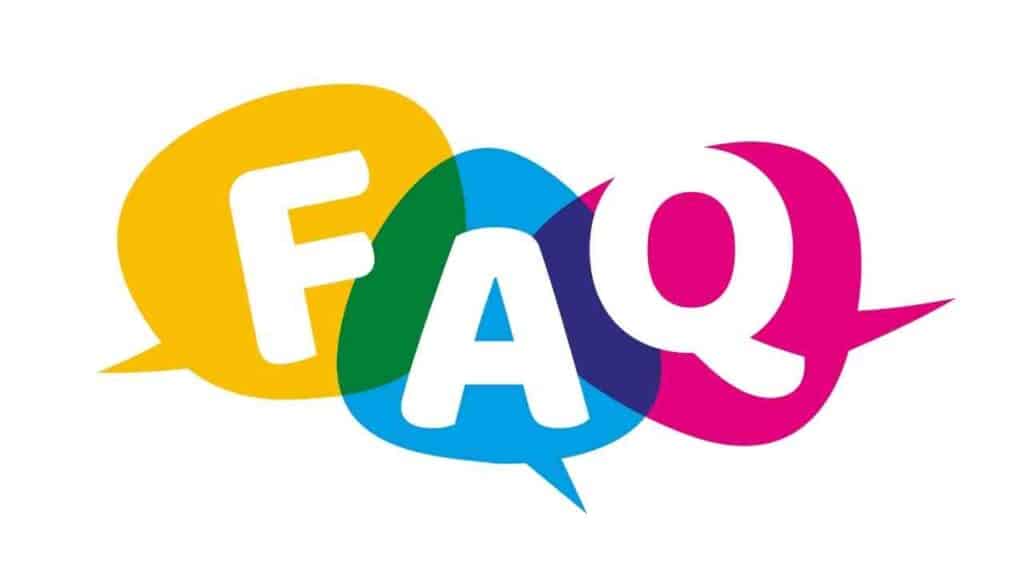
🛡️ How critical is machine learning in reinforcing the strength of our sales cycle?
In today’s hyper-competitive marketplace, machine learning is not just beneficial; it’s essential in solidifying your sales fortress! 🏰 By intelligently analyzing customer data, market trends, and internal sales processes, machine learning offers predictive insights that arm your sales team with the right tools and strategies. It’s about reinforcing your sales cycle with a shield of data-driven decisions, ensuring that every step, from targeting leads to closing deals, is optimized and fortified. This approach minimizes risks, reduces inefficiencies, and ensures your sales cycle is a well-oiled machine ready to triumph in the battlefield of market competition. 🏆
🤖 In the era of automation, how does machine learning inject efficiency into our sales processes?
Imagine transforming your sales cycle into a high-speed car that’s both fast and incredibly efficient! 🏎️ That’s what machine learning brings to the table. It automates mundane and repetitive tasks, allowing your sales team to focus on what they do best: selling. By rapidly processing and analyzing data, machine learning identifies the most promising leads, suggests the most effective engagement strategies, and even recommends the best times to follow up. It’s like having a supercharged engine in your sales vehicle, ensuring you reach your destination faster, smoother, and with less fuel (read: time and effort) than ever before. Buckle up; your sales processes are about to hit top speed! 🚀
🔎 How do we leverage machine learning for precision-targeting in our sales initiatives?
Precision is key in sales, and machine learning is your sharpshooter! 🎯 By delving into historical data and current trends, machine learning algorithms can pinpoint your ideal customer profiles with astonishing accuracy. But it goes beyond just who your customers are; it’s about knowing their behaviors, preferences, and needs. This technology empowers your sales team to tailor their approaches and solutions to match exactly what your prospects are looking for, significantly increasing the likelihood of conversion. It’s like switching from broad-spectrum lighting to a laser-focus, illuminating the path to successful sales with clarity and precision. 💡
🔄 How does machine learning continually optimize the sales cycle for sustained success?
Success in sales is not a one-time feat; it’s a continuous journey. 🛣️ Machine learning is pivotal in this aspect because it learns and evolves. With each sale, interaction, success, and setback, it gets smarter. It identifies what’s working and what’s not, suggesting tweaks and changes in real-time. For instance, if certain objections are commonly raised by prospects, machine learning will catch onto these patterns, allowing for strategies to overcome or preempt these objections in future pitches. It’s like having a very astute mentor who’s seen it all and ensures you’re perpetually one step ahead, securing not just one-off successes but sustained growth and victory. 🌱
💬 How can machine learning amplify our sales communication strategies for maximum impact?
Communication is the heartbeat of sales, and machine learning is the rhythm keeper. 🎶 It analyzes data from every customer interaction, helping understand the nuances of effective communication. Did you know that the words used, the sentiment behind the messages, and even the timing of communication can influence a sale? Machine learning does, and it utilizes this information to guide your sales team in crafting compelling messages that resonate. It’s not just about talking to your prospects; it’s about connecting with them on a deeper level. Machine learning ensures your team’s communication is not just heard but felt, fostering trust, understanding, and, ultimately, a successful sale. 🤝
- crm
- customer relationship management
- Utilizing Machine Learning to Fortify the Sales Cycle
- What is CRM Software?