Techniques to Utilize Advanced Analytics for CRM Data
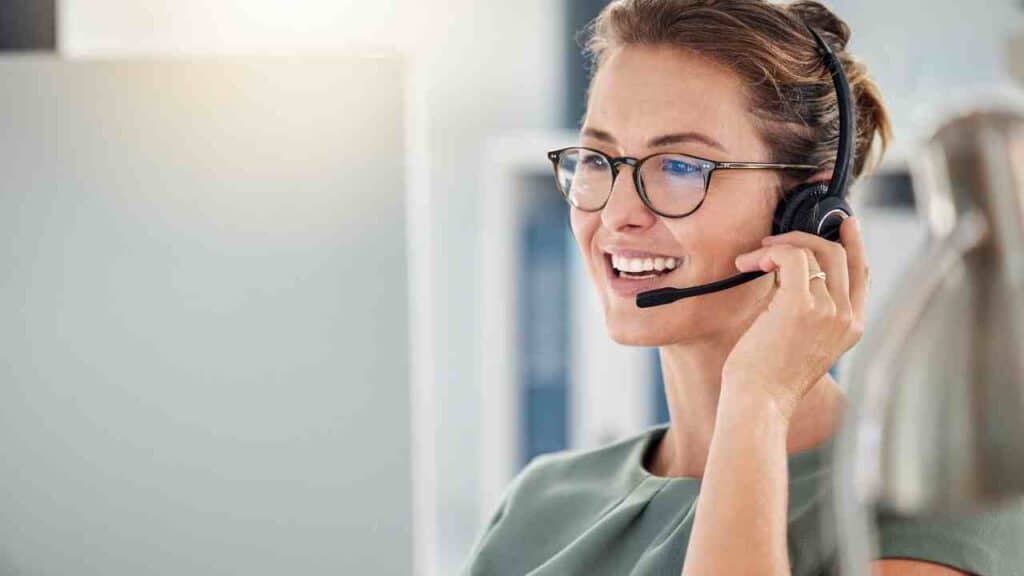
Customer Relationship Management (CRM) systems have become an integral part of modern businesses, allowing organizations to effectively manage their interactions with customers. These systems collect vast amounts of data, providing valuable insights into customer behavior, preferences, and trends. However, the true power of CRM data lies in the ability to leverage advanced analytics techniques to extract meaningful information and drive informed decision-making. In this article, we will explore various techniques to utilize advanced analytics for CRM data, along with real-world examples and case studies.
1. Data Cleaning and Preprocessing
Before diving into advanced analytics, it is crucial to ensure that the CRM data is clean and properly preprocessed. Data cleaning involves removing duplicate entries, correcting errors, and handling missing values. Preprocessing includes transforming data into a suitable format for analysis, such as converting categorical variables into numerical representations.
For example, a retail company may have a CRM system that records customer purchases. However, due to various reasons, the data may contain duplicate entries or missing values. By cleaning and preprocessing the data, the company can ensure accurate analysis and avoid misleading insights.
2. Descriptive Analytics
Descriptive analytics focuses on summarizing and visualizing CRM data to gain a better understanding of customer behavior and trends. This technique helps businesses answer questions like “Who are our most valuable customers?” or “What are the most popular products?”.
One way to utilize descriptive analytics is through customer segmentation. By dividing customers into distinct groups based on their characteristics or behaviors, businesses can tailor their marketing strategies and offerings to each segment’s specific needs. For instance, a clothing retailer may identify a segment of high-spending customers and create personalized promotions to increase their loyalty.
3. Predictive Analytics
Predictive analytics takes CRM data analysis a step further by using statistical models and machine learning algorithms to forecast future outcomes. This technique enables businesses to make data-driven predictions about customer behavior, such as purchase likelihood, churn probability, or lifetime value.
For example, a telecommunications company can use predictive analytics to identify customers at risk of churning. By analyzing historical CRM data, including factors like customer complaints, usage patterns, and contract renewal dates, the company can build a predictive model that alerts them to potential churners. This allows the company to take proactive measures, such as offering personalized retention offers, to prevent customer attrition.
4. Prescriptive Analytics
Prescriptive analytics goes beyond predicting future outcomes and provides recommendations on the best course of action. This technique leverages advanced algorithms and optimization models to suggest optimal strategies for maximizing customer satisfaction, revenue, or other key performance indicators.
For instance, an e-commerce platform can utilize prescriptive analytics to optimize its pricing strategy. By analyzing CRM data, market trends, and competitor prices, the platform can determine the optimal price points for different products to maximize revenue while remaining competitive.
5. Sentiment Analysis
Sentiment analysis is a technique that uses natural language processing and machine learning to analyze customer feedback, such as reviews, social media posts, or support tickets. By understanding customer sentiment, businesses can gain insights into customer satisfaction, identify areas for improvement, and detect emerging trends.
For example, a hotel chain can perform sentiment analysis on customer reviews to identify common complaints or areas of praise. This information can then be used to improve service quality, address customer concerns, and enhance the overall customer experience.
6. Case Study: XYZ Bank
Let’s take a look at a real-world example of how advanced analytics techniques were utilized to improve CRM data analysis. XYZ Bank, a leading financial institution, wanted to enhance its customer retention efforts. By leveraging advanced analytics, they were able to identify key factors contributing to customer churn and develop targeted retention strategies.
First, XYZ Bank performed data cleaning and preprocessing to ensure the accuracy and consistency of their CRM data. They then applied predictive analytics techniques to build a churn prediction model. By analyzing historical customer data, including transaction history, account activity, and customer demographics, they identified patterns and indicators of potential churn.
Based on the insights gained from the churn prediction model, XYZ Bank implemented personalized retention strategies. For example, they offered tailored promotions and discounts to customers identified as high-risk churners. They also proactively reached out to customers with low engagement levels to understand their concerns and provide personalized solutions.
The results were impressive. XYZ Bank saw a significant reduction in customer churn rates and an increase in customer satisfaction. By utilizing advanced analytics techniques, they were able to make data-driven decisions and improve their CRM data analysis capabilities.
Utilizing advanced analytics techniques for CRM data can provide businesses with valuable insights into customer behavior, preferences, and trends. By cleaning and preprocessing the data, businesses can ensure accurate analysis. Descriptive analytics helps in summarizing and visualizing CRM data, while predictive analytics enables businesses to make data-driven predictions about customer behavior. Prescriptive analytics goes a step further by providing recommendations on the best course of action. Sentiment analysis helps in understanding customer sentiment and improving the overall customer experience.
Real-world examples, such as XYZ Bank, demonstrate the effectiveness of advanced analytics in improving CRM data analysis and driving informed decision-making. By leveraging these techniques, businesses can gain a competitive edge, enhance customer satisfaction, and drive revenue growth.
Visit https://SaasExpert.ca – Your All-In-One Sales and Marketing Platform for small businesses, agency owners, and marketers.
Frequently asked questions about Techniques to Utilize Advanced Analytics for CRM Data.
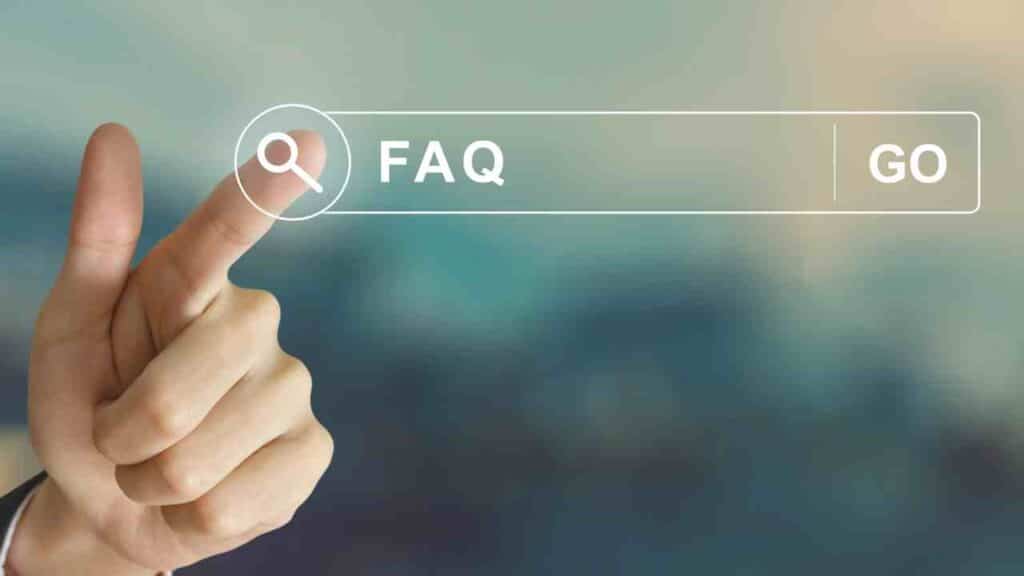
What types of insights can advanced analytics offer for my CRM data? 🔍
Terrific question! Advanced analytics can turn your raw CRM data into actionable insights that can transform how you do business. For example, you can use predictive analytics to forecast future buying behavior, cluster analysis to segment your customer base into meaningful groups, or even natural language processing to analyze customer feedback. These insights can inform everything from product development to personalized marketing strategies. It’s all about using data to make smarter decisions.
How can I get started with applying advanced analytics to my CRM? 🛠️
Ah, the all-important first step! First, identify what you want to achieve—be it higher sales, better customer engagement, or more effective marketing campaigns. Then decide on the metrics that will help you measure these goals. Once you’ve got that down, it’s time to pick the right analytical tools that can help you crunch those numbers. This might be built-in features of your CRM or specialized third-party analytics tools. Lastly, you’ll want to train your team on how to interpret the data and implement data-driven actions.
How often should I be analyzing my CRM data? ⏰
Excellent question! The frequency of analysis depends on your business goals and the volume of data you’re handling. If you’re tracking metrics that change frequently, such as website visits or short-term campaign results, you’ll want to analyze the data daily or weekly. For long-term goals like customer lifetime value or seasonal trends, monthly or even quarterly analyses might suffice. The key is to strike a balance between being informed and not getting overwhelmed by the data.
What challenges might I face when applying advanced analytics to CRM data? 🏋️♀️
Ah, no journey is without its hurdles! One of the main challenges is ensuring data quality. Your analytics are only as good as the data feeding them. Inaccurate or incomplete data can lead to misleading insights. Another challenge is the complexity of analytics. It’s crucial to have a team that understands both the tools and the business context to accurately interpret results. Finally, let’s not forget about data security. As you collect and analyze more sensitive customer data, the responsibility to protect this data increases exponentially.
Can advanced analytics help improve customer service? 🤝
Absolutely, they can be a game-changer! By analyzing historical interactions, response times, and customer feedback, you can identify areas for improvement in your customer service. For instance, if the data shows that customers frequently ask the same questions, you could implement a chatbot to handle these queries, freeing up human agents to deal with more complex issues. Analytics can also help you identify at-risk customers, allowing you to proactively address their concerns before they escalate.
There you go, your top 5 questions on techniques to utilize advanced analytics for CRM data answered! Analytics can truly elevate your CRM strategy, turning you into not just a data-driven business, but an insight-driven one! 🎯
- crm
- customer relationship management
- Techniques to Utilize Advanced Analytics for CRM Data
- What is CRM Software?