Optimizing Sales Cycle Processes with Machine Learning
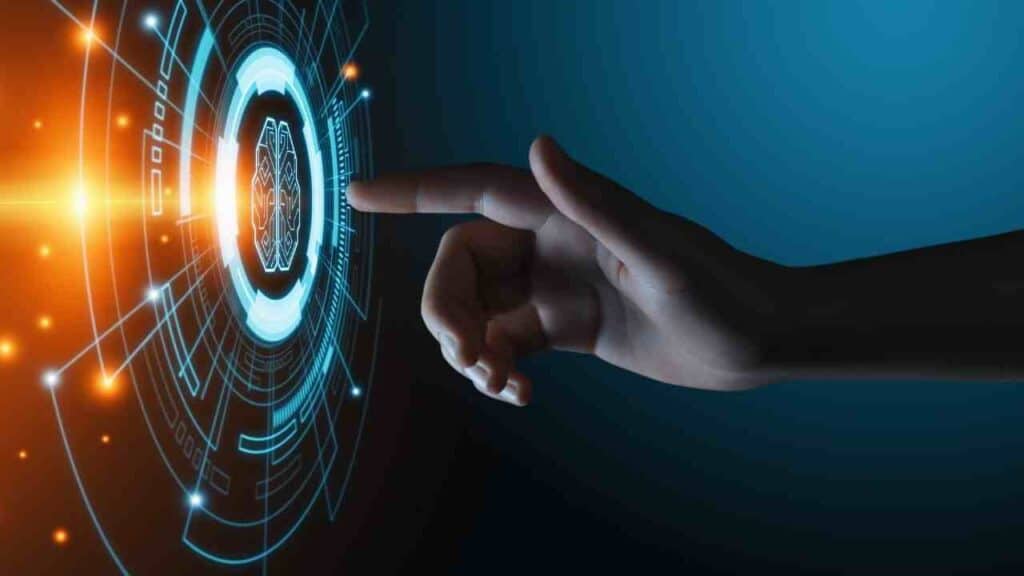
Machine learning has revolutionized various industries, and sales is no exception. With the ability to analyze vast amounts of data and make accurate predictions, machine learning algorithms can optimize sales cycle processes, leading to increased efficiency, higher conversion rates, and improved customer satisfaction. In this article, we will explore how machine learning can be leveraged to enhance sales cycle processes and provide valuable insights for businesses.
The Sales Cycle: An Overview
Before delving into the role of machine learning in optimizing sales cycle processes, let’s first understand what the sales cycle entails. The sales cycle refers to the series of steps a customer goes through before making a purchase. It typically includes prospecting, lead generation, lead qualification, product demonstration, negotiation, and closing the deal.
Traditionally, sales teams have relied on manual processes and intuition to navigate the sales cycle. However, this approach is time-consuming, prone to human error, and often lacks data-driven insights. Machine learning can address these challenges by automating and optimizing various stages of the sales cycle.
1. Prospecting and Lead Generation
Prospecting and lead generation are crucial stages in the sales cycle, as they involve identifying potential customers and gathering relevant information about them. Machine learning algorithms can analyze vast amounts of data from various sources, such as social media, online platforms, and customer databases, to identify potential leads with a high likelihood of conversion.
For example, a machine learning algorithm can analyze customer behavior patterns and demographic data to identify individuals who are more likely to be interested in a particular product or service. By leveraging this information, sales teams can focus their efforts on leads that have a higher probability of conversion, saving time and resources.
2. Lead Qualification
Lead qualification involves determining whether a lead meets the criteria to be considered a potential customer. Machine learning algorithms can automate this process by analyzing various factors, such as lead demographics, past purchase history, and engagement with marketing materials.
By leveraging machine learning, businesses can develop lead scoring models that assign a numerical value to each lead based on their likelihood of conversion. This allows sales teams to prioritize leads and focus their efforts on those with a higher score, increasing the chances of closing deals.
3. Product Demonstration and Personalization
Product demonstrations play a crucial role in convincing potential customers of the value and benefits of a product or service. Machine learning can enhance this stage by providing personalized product recommendations and tailored demonstrations based on the customer’s preferences and past behavior.
For instance, machine learning algorithms can analyze customer data to understand their preferences, purchase history, and browsing behavior. This information can then be used to recommend specific products or features that are likely to resonate with the customer, increasing the chances of a successful product demonstration and ultimately closing the deal.
4. Negotiation and Pricing
Negotiation and pricing are often complex and time-consuming processes in the sales cycle. Machine learning algorithms can analyze historical sales data, market trends, and customer preferences to provide valuable insights for pricing and negotiation strategies.
By leveraging machine learning, businesses can develop dynamic pricing models that take into account various factors, such as customer demand, competitor pricing, and product availability. This allows sales teams to optimize pricing strategies and negotiate deals more effectively, leading to increased customer satisfaction and higher conversion rates.
5. Closing the Deal and Customer Retention
Closing the deal is the final stage of the sales cycle, but it is not the end of the customer journey. Machine learning can play a crucial role in customer retention by analyzing customer data and providing insights for personalized post-sales engagement.
For example, machine learning algorithms can analyze customer behavior and purchase history to identify potential upsell or cross-sell opportunities. By leveraging this information, businesses can tailor their post-sales communication and offers to meet the specific needs and preferences of each customer, increasing customer satisfaction and loyalty.
Case Study: Optimizing Sales Cycle with Machine Learning
One real-life example of how machine learning can optimize sales cycle processes is the case of a software-as-a-service (SaaS) company. The company used machine learning algorithms to analyze customer data and identify potential upsell opportunities.
By analyzing customer behavior patterns, usage data, and customer support interactions, the machine learning algorithm identified customers who were likely to benefit from additional features or upgrades. The sales team then used this information to tailor their upsell offers and communication, resulting in a significant increase in upsell revenue and customer satisfaction.
The Benefits of Optimizing Sales Cycle Processes with Machine Learning
By leveraging machine learning to optimize sales cycle processes, businesses can benefit in several ways:
- Increased efficiency: Machine learning automates time-consuming tasks, allowing sales teams to focus on high-value activities.
- Higher conversion rates: By prioritizing leads with a higher likelihood of conversion, businesses can increase their conversion rates and revenue.
- Improved customer satisfaction: Personalized product recommendations and tailored communication based on customer preferences lead to higher customer satisfaction and loyalty.
- Data-driven insights: Machine learning provides valuable insights based on data analysis, enabling businesses to make informed decisions and optimize their sales strategies.
Machine learning has the potential to revolutionize sales cycle processes by automating and optimizing various stages of the sales journey. From prospecting and lead generation to closing the deal and customer retention, machine learning algorithms can provide valuable insights and enhance efficiency, conversion rates, and customer satisfaction.
By leveraging machine learning, businesses can gain a competitive edge in today’s fast-paced sales environment. With the ability to analyze vast amounts of data and make accurate predictions, machine learning algorithms can provide valuable insights that drive sales success.
Optimize your sales cycle processes with machine learning today. Visit https://SaasExpert.ca – Your All-In-One Sales and Marketing Platform for small businesses, agency owners, and marketers.
Frequently asked questions about Optimizing Sales Cycle Processes with Machine Learning
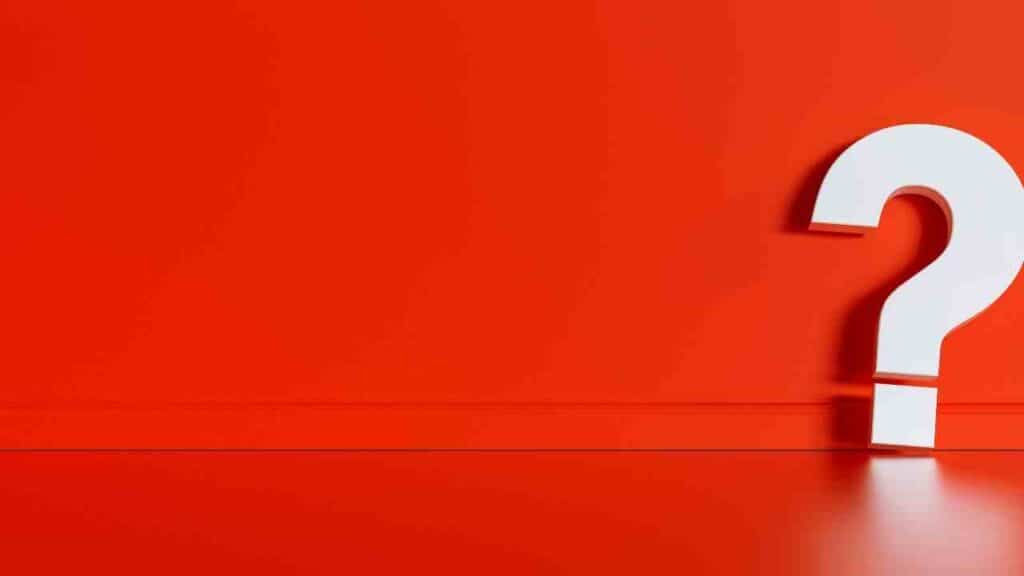
🚀 How does machine learning accelerate the lead generation process in sales?
Get ready for a speed boost in your lead generation like never before! 🌪️ Machine learning takes the driver’s seat by implementing advanced algorithms that swiftly sift through massive data sets, identifying potential leads based on online behavior, purchase history, and more. But it doesn’t stop there! It continuously learns from these interactions, refining strategies to capture leads faster and more efficiently. This means your sales team can wave goodbye to cold calling and generic pitches. Instead, they’ll engage pre-qualified leads, ready for the next step in the sales journey. It’s about hitting the accelerator and making every sales opportunity count! 🏁
💡 Can machine learning really illuminate the path to more successful sales strategies?
Absolutely, it’s like having a strategic oracle on your side! 🔮 Machine learning shines a light on the successful paths by analyzing past strategies, market trends, and customer behavior. Imagine knowing precisely what worked, what didn’t, and most importantly, why. Machine learning provides these insights, empowering your sales team to craft strategies that resonate deeply with potential clients. It’s about turning every ‘no’ or ‘maybe’ into a data point that guides your next ‘yes.’ With machine learning, your sales strategy evolves from a game of guesswork to a science of success. 🎯
🕵️♂️ How does machine learning enhance customer insight for better sales engagement?
Dive deeper into the minds of your customers with machine learning! 🧠 By analyzing data points from various interactions (like website visits, social media engagement, and past communications), machine learning builds a comprehensive understanding of your customers’ needs and preferences. It’s like having a private detective that gathers clues to complete the picture of what your customers truly desire. This insight is crucial, allowing your sales team to engage prospects with the right message, at the right time, through the right channel. Personalized, impactful engagement? That’s the power of enhanced customer insight! 💥
🎛 In what ways does machine learning optimize the decision-making process in our sales cycle?
Welcome to decision-making at its finest, where machine learning is your master tuner! 🎛 It works behind the scenes, processing a plethora of data from various sources, and presents it in an actionable format. From determining the best timing for follow-ups to prioritizing high-value leads, machine learning eliminates the guesswork. It allows your sales team to make quick, informed decisions, aligning their actions with the most promising outcomes. Imagine sidestepping the trial-and-error approach, moving straight to choices that propel the sales journey forward. That’s strategic, optimized decision-making in action! ♟️
🧲 How effective is machine learning in strengthening customer retention within the sales cycle?
Think of machine learning as a magnet that not only attracts but also keeps your customers drawn to you! 🧲 Post-sale, machine learning analyzes customer feedback, support interactions, and product usage to predict customer satisfaction levels. Not stopping at just insights, it suggests actions to keep your customers’ loyalty intact — be it a well-timed check-in, a special offer, or a personalized note thanking them for their business. By proactively addressing concerns and enhancing satisfaction, machine learning ensures that your sales cycle doesn’t end at the point of sale. Instead, it evolves into a cycle of sustained loyalty and recurring business. 🔄🏅
- crm
- customer relationship management
- Optimizing Sales Cycle Processes with Machine Learning
- What is CRM Software?