How can Machine Learning be used to increase sales cycle?
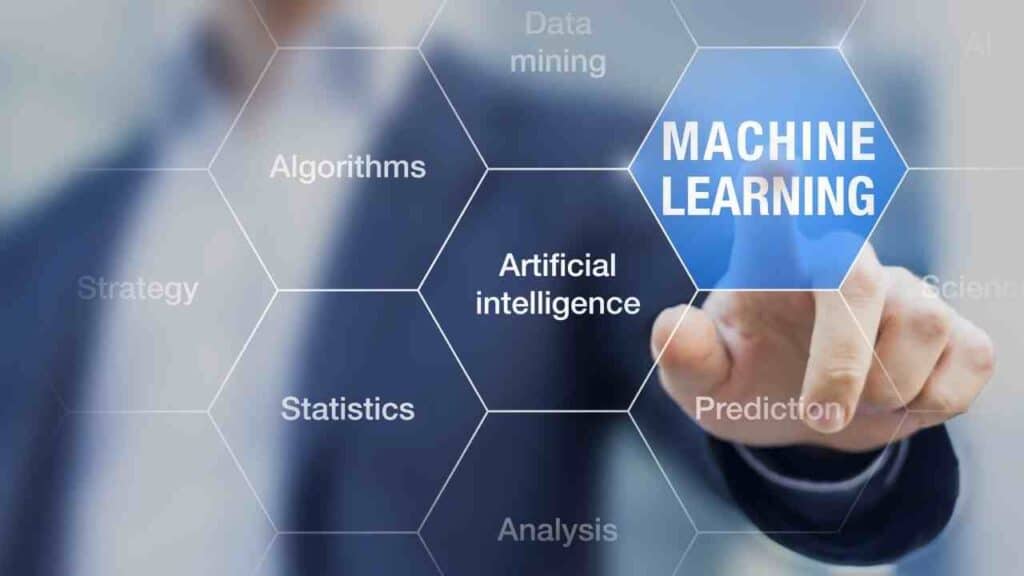
Machine learning, a subset of artificial intelligence, has been making waves across various industries. From healthcare to finance, machine learning algorithms have proven to be powerful tools for analyzing vast amounts of data and making accurate predictions. One area where machine learning has the potential to make a significant impact is the sales cycle. By leveraging machine learning techniques, businesses can streamline their sales processes, improve customer targeting, and ultimately increase sales. In this article, we will explore how machine learning can be used to enhance the sales cycle and drive business growth.
Understanding the Sales Cycle
Before delving into the ways machine learning can optimize the sales cycle, it is essential to have a clear understanding of what the sales cycle entails. The sales cycle refers to the series of steps a customer goes through before making a purchase. It typically includes stages such as lead generation, lead qualification, product demonstration, negotiation, and closing the deal. Each stage requires careful planning, effective communication, and a deep understanding of the customer’s needs and preferences.
Enhancing Lead Generation
Lead generation is the first step in the sales cycle, and it involves identifying potential customers who have shown interest in a product or service. Traditionally, businesses rely on manual methods such as cold calling, email campaigns, and trade shows to generate leads. However, these methods can be time-consuming and often yield low-quality leads.
Machine learning algorithms can significantly improve lead generation by analyzing vast amounts of data to identify patterns and predict customer behavior. By leveraging historical data, machine learning models can identify the characteristics of high-quality leads and target similar individuals or companies. For example, a machine learning algorithm can analyze customer data to identify common traits among customers who have made a purchase in the past, such as demographics, online behavior, and purchase history. This information can then be used to target potential customers who exhibit similar characteristics, increasing the chances of converting them into paying customers.
Personalized Customer Engagement
Once leads have been generated, the next step is to engage with them in a personalized and meaningful way. Traditional sales approaches often involve generic sales pitches that may not resonate with individual customers. This lack of personalization can lead to disengagement and lost opportunities.
Machine learning can help businesses personalize their customer engagement by analyzing customer data and generating insights. By leveraging machine learning algorithms, businesses can gain a deeper understanding of their customers’ preferences, needs, and pain points. For example, by analyzing customer interactions with a website or app, machine learning models can identify patterns and make recommendations for personalized product offerings or content. This level of personalization can significantly enhance the customer experience and increase the likelihood of a successful sale.
Optimizing Sales Forecasting
Accurate sales forecasting is crucial for businesses to make informed decisions and allocate resources effectively. Traditional sales forecasting methods often rely on historical data and manual analysis, which can be time-consuming and prone to human error.
Machine learning algorithms can revolutionize sales forecasting by analyzing vast amounts of data and identifying patterns that humans may overlook. By considering various factors such as historical sales data, market trends, and customer behavior, machine learning models can generate accurate sales forecasts. This enables businesses to make data-driven decisions, optimize inventory management, and allocate resources more effectively.
Improving Sales Team Performance
The performance of a sales team plays a crucial role in the success of a business. Identifying areas for improvement and providing targeted training can significantly enhance the performance of individual sales representatives.
Machine learning can help businesses identify patterns and trends in sales team performance by analyzing various data points such as call recordings, email interactions, and customer feedback. By leveraging machine learning algorithms, businesses can identify areas where sales representatives may be struggling and provide targeted training or coaching to address these issues. This data-driven approach to sales team management can lead to improved performance, increased sales, and higher customer satisfaction.
Case Study: XYZ Company
One real-world example of how machine learning can increase sales cycle efficiency is XYZ Company, a software-as-a-service (SaaS) provider. XYZ Company implemented a machine learning algorithm to analyze customer data and identify potential upsell opportunities. By analyzing customer usage patterns, the algorithm identified customers who were likely to benefit from additional features or upgrades. This allowed the sales team to target these customers with personalized offers, resulting in a significant increase in upsell revenue and overall customer satisfaction.
Machine learning has the potential to revolutionize the sales cycle by enhancing lead generation, personalizing customer engagement, optimizing sales forecasting, and improving sales team performance. By leveraging machine learning algorithms, businesses can gain valuable insights from vast amounts of data, enabling them to make data-driven decisions and drive business growth. As technology continues to advance, the integration of machine learning into sales processes will become increasingly important for businesses looking to stay competitive in today’s fast-paced market.
Visit https://SaasExpert.ca – Your All-In-One Sales and Marketing Platform for small businesses, agency owners, and marketers.
Frequently asked questions about How Machine Learning Can Revolutionize the Sales Cycle.
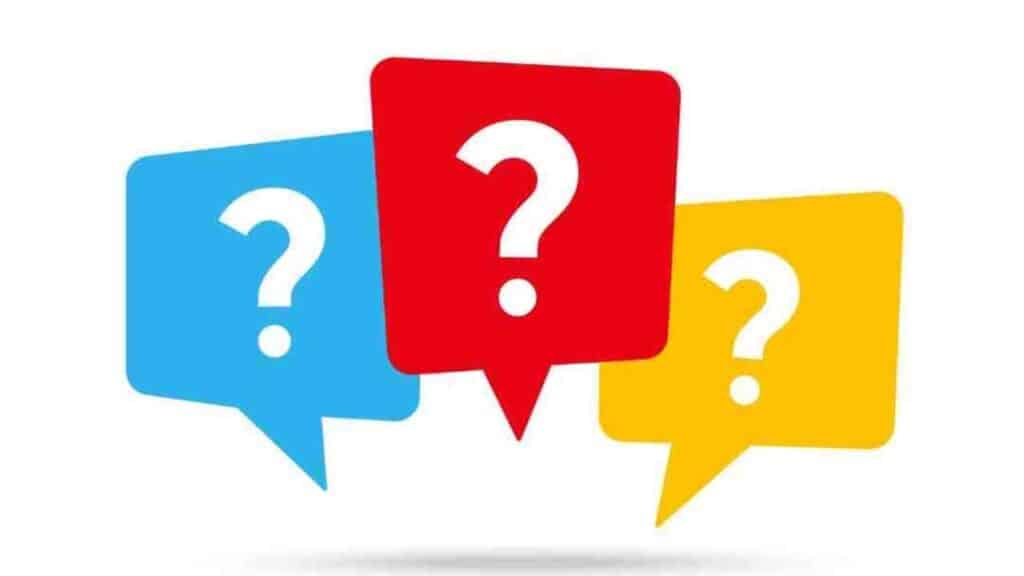
🚀 How does machine learning bring a paradigm shift in understanding customer behavior during the sales cycle?
Absolutely transformative! 🌟 Machine learning dives deep into customer data, analyzing patterns that can be almost invisible to the naked eye. Traditional sales methods involve a lot of guesswork and assumptions about customer behavior. But now, with machine learning, sales teams are equipped with profound insights. By continually learning from customer interaction data, purchasing histories, and even external information (like market trends), ML algorithms can predict customer behavior, preferences, and potential pain points with astounding accuracy. This enables sales professionals to personalize engagement strategies, align sales pitches with actual customer needs, and build rapport at an unprecedented scale, effectively revolutionizing the customer journey from initial contact to closing. 🏁
💡 Can machine learning truly optimize sales forecasting and pipeline management?
Indeed, it’s a game-changer! 🔥 Traditional sales forecasting often relies on historical data and sales rep experience, both subjective and prone to inaccuracies. Enter machine learning, the superhero in this scenario! It takes forecasting to the next level by analyzing vast datasets in real time, identifying complex patterns, and even accounting for unforeseen market dynamics. For pipeline management, machine learning provides predictive scoring, letting sales reps focus on leads that are more likely to convert, thereby optimizing effort and resource allocation. Essentially, machine learning takes the guesswork out of forecasting and pipeline management, replacing it with data-driven insights that continually improve over time, ensuring your sales team is working smarter, not harder! 🎯
🧠 How does machine learning enhance decision-making for sales strategies?
Think of it as your analytical powerhouse! 💪 Machine learning isn’t just about data; it’s about actionable insights. By sifting through customer interactions, feedback, market trends, and competitor strategies, it provides a holistic view far beyond human capacity. Sales teams can rely on ML to identify which strategies worked (or flopped 📉), why certain deals took longer to close, and what factors influenced customer decisions. Armed with this knowledge, sales strategies can be adjusted and optimized, focusing on productive avenues and innovative approaches tailored to meet evolving customer expectations. Decision-making becomes faster, data-backed, and aligned with real-world feedback, ensuring your sales strategy is a living, evolving entity, not a static plan. 🚀
🔍 How does machine learning streamline lead qualification in the sales cycle?
It’s like having a crystal ball! 🔮 Traditional lead qualification can be a drain, with sales teams spending precious time and energy sifting through leads. Machine learning transforms this by swiftly analyzing lead data (from communication, engagement, social media interactions, etc.) and scoring leads based on their likelihood to convert. But that’s not all! It also provides insights into why a lead is qualified or not, allowing for more nuanced follow-up strategies. Sales reps can engage high-quality leads with the right approach, at the right time, with the right product or solution, significantly increasing conversion rates and ensuring no opportunity goes unnoticed. 🌈
🌐 In what ways does machine learning contribute to enhancing post-sale customer relationships?
Beyond closing deals, it’s the golden key to customer loyalty! 🗝️ Post-sale relationships are critical for ensuring repeat business and positive referrals. Machine learning helps monitor customer satisfaction through feedback analysis, support interactions, and product engagement, highlighting areas needing immediate attention. 🚨 By predicting customer needs, it allows for timely check-ins, personalized offers, and appropriate support, fostering a sense of trust and personalized care. Additionally, it identifies up-sell or cross-sell opportunities, helping sales teams understand when to pitch an additional product or service. With machine learning, post-sale isn’t an afterthought but an opportunity to solidify relationships and create brand advocates. 💖
- crm
- customer relationship management
- How Machine Learning Can Revolutionize the Sales Cycle
- What is CRM Software?